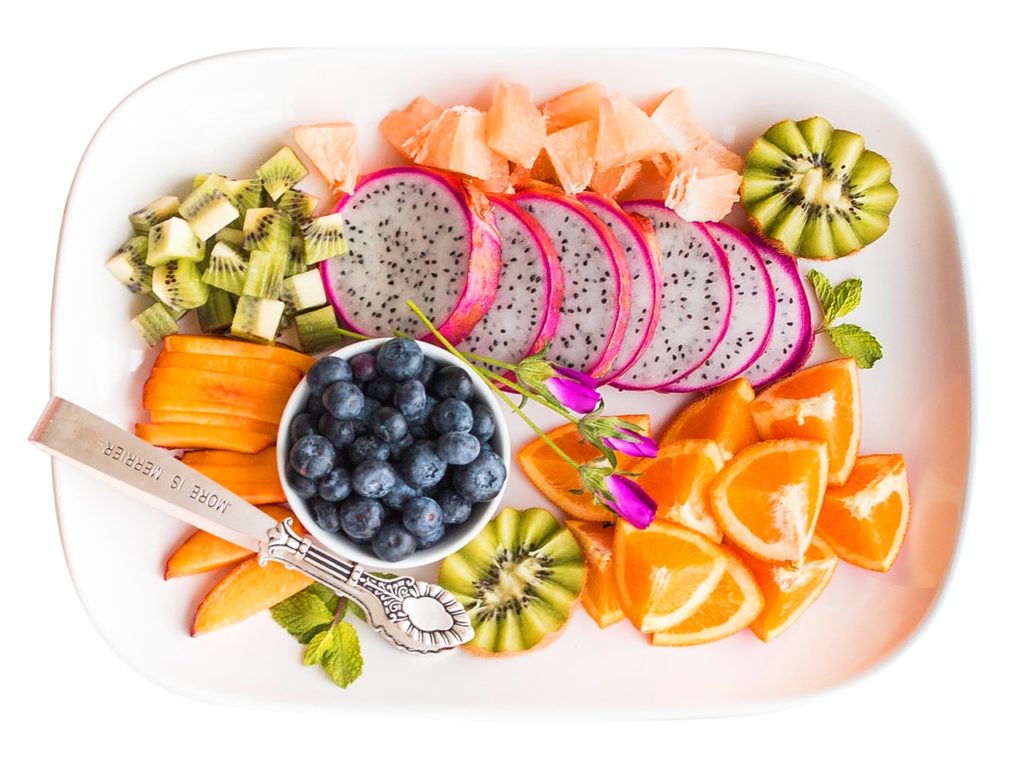
Human learning and machine learning are very similar. They are similar in the sense that both involve learning, the process of understanding and creating new knowledge.
If we, humans, can learn from our teachers through books, theorems, definitions, and examples, etc., machines can also learn from us through data. If our teachers can guide us, tell us what is right, what is wrong, which is an apple and which is an orange, we can, in turn, teach our machines the same with labeled data. This is supervised learning.
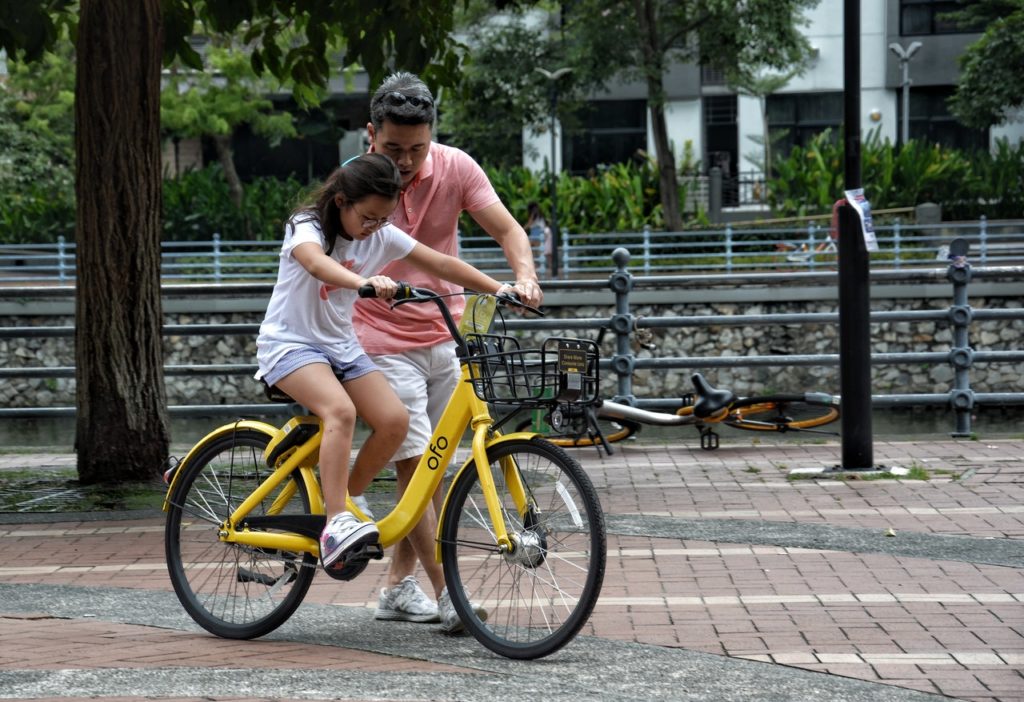
If we are not able to come to school, we don’t have teachers, then we have to learn by ourselves. We will not be able to differentiate rights from wrongs, because we are not taught. Instead, not being guided makes us go to a novel, unexpected direction, and hence create new values. Unsupervised learning of machines is the same. As the machine doesn’t have labeled data, it uses unlabeled data and its role is to devise new knowledge.
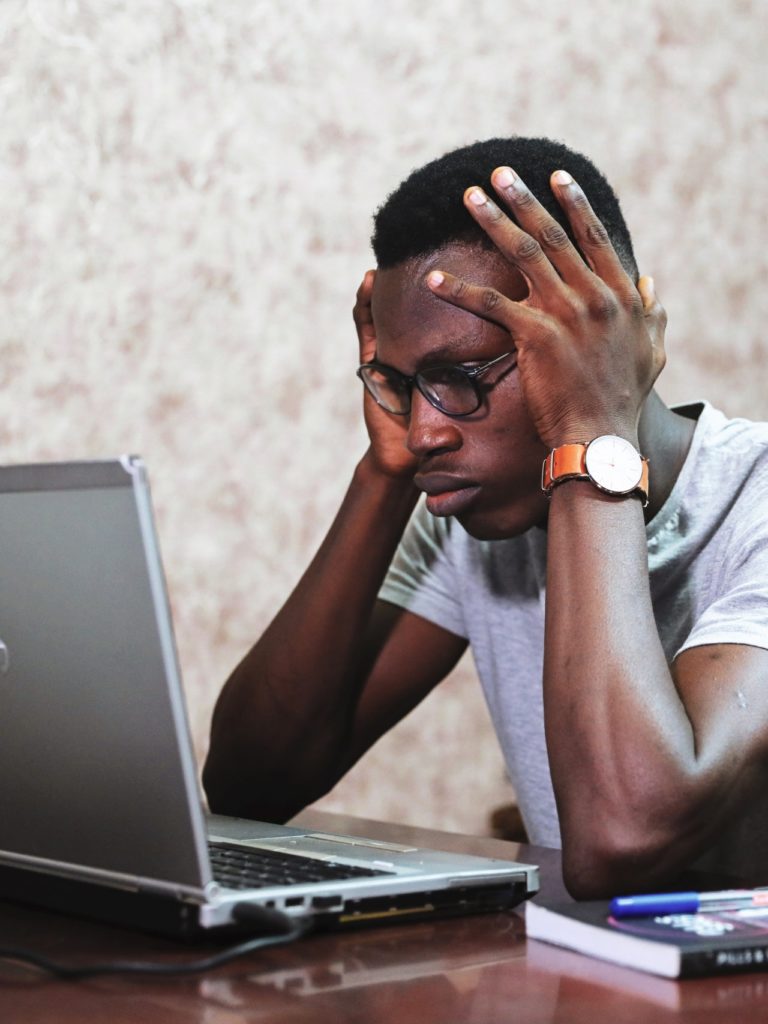
The last type we are supposed to think about is reinforcement learning. Imagine you are a strict parent, you want your child to get things done on his own. When your child does something right, you praise him, but he will get punished when doing things wrongly. You never teach him how to handle things, but do let him know if his action is good or bad. Machine’s reinforcement learning is just like that. Your machine gets unlabeled data (same as unsupervised learning), but when it outputs a prediction, you tell it if its output is good or bad, right or wrong.
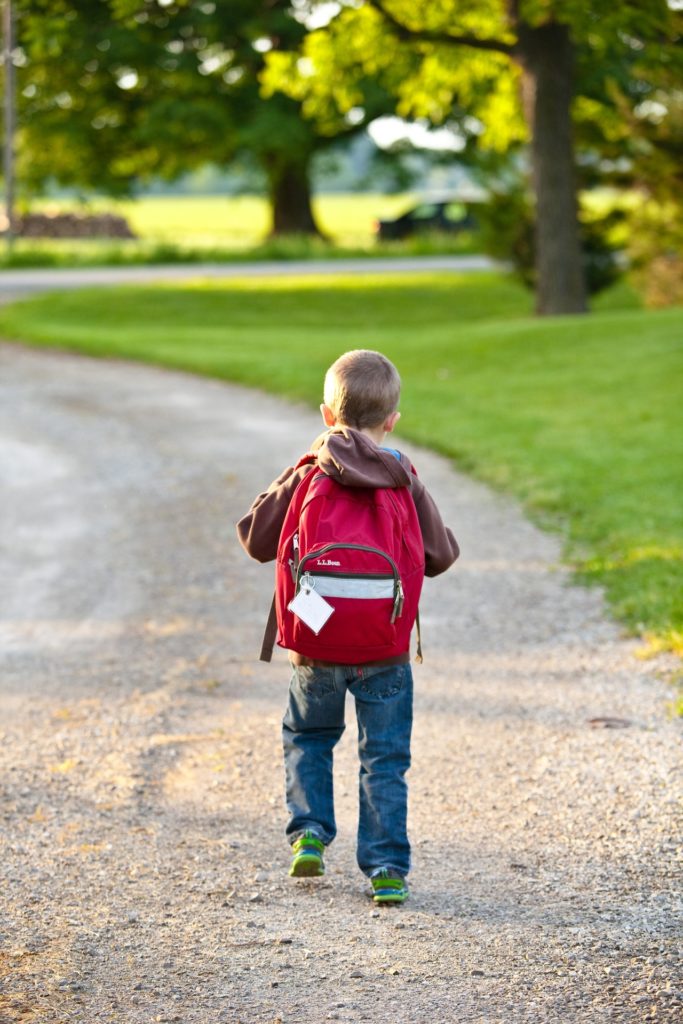